How Do We Foster Inclusive and Collaborative AI Policy Research?
Tags
On the 14th and 15th of November, the AI Policy Lab at Umeå University and the Mila-Quebec AI institute convened a collaborative summit on AI research and policy. The summit, which was held in Stockholm, brought together several AI research and policy experts with a focus on fostering collaboration in AI governance across academia, think tanks, civil society, and government. GCG researcher, Ayantola Alayande, reflects on key discussion points from the summit.
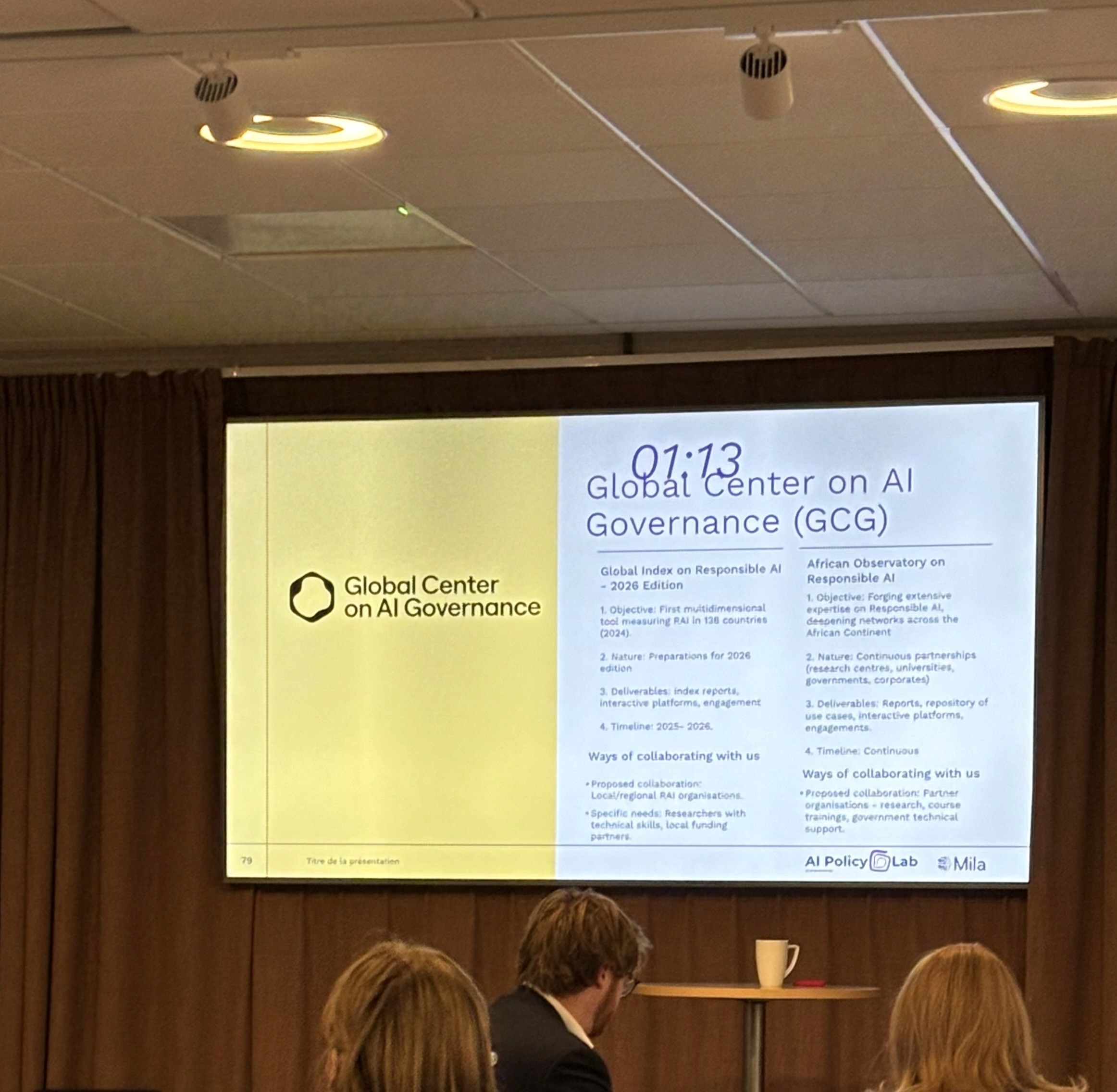
Why ‘Collaborative’ AI Policy Research?
With AI research being driven by its focus on the fundamental science of machine learning and policy being influenced by the socio-economic outcomes of AI systems, there is a seeming disconnect between AI research and policymaking for AI. Scientific progress in AI without considerations for its societal implications risks reproducing existing harms; at the same time, policy without adequate scientific and technical insight about how AI works only leads to discordant or excessive regulation and regulatory arbitrage. As such, a key focus of the AI Policy Summit was to explore ways of embedding policy principles in AI research and vice versa.
The first crucial step in doing this was to approach things differently. One unique feature of the Summit was its structure: representatives from various organisations presented projects they were working on and areas where collaboration was needed. This collaborative approach marked a shift from the conventional approach to a problem-solving approach that aims to reduce duplicity of efforts.
Starting Within: How Can Researchers Ensure a Broader and Inclusive Approach to AI Research?
As AI systems evolve, the conventional understanding of mathematics and computing science as the dominant lenses in AI research is outdated. In today’s research community, scholars from the arts, humanities, social, and health sciences are making novel contributions on the technical and ethical fronts of AI. Working together not only ensures that researchers benefit from the diverse range of perspectives in the room, it also limits potential blind spots that may arrive from being accustomed to only one disciplinary approach.
Aside from interdisciplinarity, connecting fundamental science with applied AI research is key to avoiding a misalignment of needs and values. For example, developers of healthcareAI systems must have a robust awareness of medical or clinical principles. Conversely, beyond the academic incentive to publish, communicating research findings to a wider audience is crucial for harnessing basic AI research for real world application and informing critical publics.
Equally crucial is international collaboration – especially between Global North and South researchers. Not only does this accelerate capacity building and knowledge sharing across borders, it is also a significant step towards achieving equitable AI futures.
Moreover, it is important to recognise the role that research outside of conventional research labs or university departments play in advancing AI governance. The private sector, government and civil society sectors are increasingly engaging in action-based, community-led AI governance research which academia can tap into. Relatedly, AI governance is not only done by governments; results from the Global Index on Responsible AI (GIRAI) 2024 shows that non-state actors like civil society organisations (CSOs) and transnational networks are actively shaping the direction of AI governance – especially in African countries.
Finally, the biggest gap identified in the summit is the disconnect between researchers and policymakers. Too often, AI policy summits exclude legislators and decision-makers, resulting in research that does not translate to actionable policy. Closing this gap involves recognising that AI policy research is incomplete without inputs from government stakeholders. This is an area of controversy in the research community as it raises questions about the neutrality and objectivity of research. However, a two-way approach is crucial, wherein academics invite government representatives to policy research summits and also actively seek collaborations through participation in government-led gatherings or communicating research in policymakers’ language.
An Agenda for the Policy-Research Collaboration
Enabling effective science and policy interaction in AI Governance requires both a value-led approach and actionable steps. Below are some considerations for researchers:
Considerations for research
Embedding AI researchers within governments: Positioning AI researchers within policymaking institutions helps to improve policymakers’ understanding of how AI works and ensures that governments appropriately leverage AI for evidence-based decision-making.
Writing accessible policy briefs: Researchers should focus on creating concise, impactful documents tailored for policymakers, addressing the need for clear communication across disciplines. Perhaps providing researchers with training in this area is a crucial part of AI policy research.
Developing a repository that provides resources on AI research for policymakers, but also on policy for AI researchers. This needs to be both ways – AI researchers need to be able to draw from a policy repository and vice versa. It is often the case that research communities do well with keeping policy observatories, but policymakers do not adequately synthesise academic research for government’s use. Such repositories might include a collection of digital policies, a mapping of legislations, use cases, etc.
Considerations for Policy (Government)
Defining values: The central pursuit of AI governance is ensuring equitable distribution of AI’s potential and minimising its risks. To achieve this, innovation must be aligned with democratic values. This includes setting an underpinning philosophy about AI use in the public sector – for example, guidelines for AI procurement in critical sectors like healthcare.
The risk-benefit matrix: Similar to the concept of defining values, this means that experts should recommend AI systems only when benefits outweigh the risks. While AI offers immense potential for evidence-generation and improving public service delivery, there are situations where AI is in fact not needed, recognising that a techno-solutionist approach to public policy problems often crowds out other effective non-tech alternatives.
Minimum acceptable governance standards: The borderless nature of AI systems means it is easier for technology companies to bypass local legal frameworks or exploit differences in regulatory standards to gain competitive advantage. Setting minimum acceptable standards for AI governance, akin to frameworks in other sectors like finance or aviation, helps reduce the risk of regulatory arbitrage.
The Task Ahead
As AI systems evolve, so must our approach to its governance and policy adapt to its progress. This task requires creativity, flexibility, and a collective effort that can only happen by fostering a ‘community of practice’. The first task in strengthening collaboration between AI research and policy is to map out possible partnership pathways. There are many pathways for research to influence policy, but two key ones are discussed in the summit: (i) training scientists to do more policy-targeted, concise type of work on AI research (ii) fostering partnerships through co-organising activities with government officials.
The second task is to think about the scope of this community of practice. Should this happen at the domestic, national, regional or international level? When thinking about international collaboration, the earlier point about defining values is crucial to defining the scope of inclusion. For example, international collaboration may want to exclude contexts with a history of authoritarianism, where strengthening AI systems only further reinforces digital repression.
Finally, and perhaps most importantly, is defining the mode of engagement. One key challenge is that stakeholders on both ends of the research and policy spectrum already have so much to take on; hence, engaging in a way that does not strain already limited resources is crucial. This might include tapping into existing resources such as university research fellowships and policy exchange programmes rather than establishing new strands of work. For us at the Global Center on AI Governance, this means creatively scaling our recent AI ethics certification courses partnership with the University of Cape Town and the University of Pretoria to bring in government experts, and delivering similar training to regulatory agencies across African countries.
Author: Ayantola Alayande
Acknowledgement:
This analysis is based on research funded by the International Development Research Centre (IDRC) and UK International Development under the AI4D program, as part of the African Observatory on Responsible AI.